Financial markets are constantly changing and have a tendency to be extremely volatile. However, there are periods where market conditions remain fairly steady and being able to identify these conditions allows investors to expose themselves to the right assets that tend to perform in that given condition.
Modelling these conditions using machine learning algorithms like Gaussian Mixture Models(GMM) or more exotic algorithms which combine multiple ML models are extremely useful in identifying the changing market conditions which enables us to better manage tail-risk.
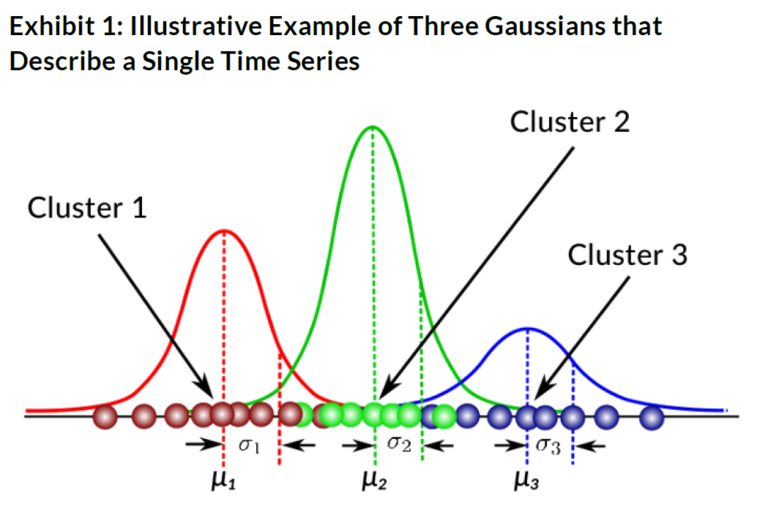
In order to understand how a portfolio might react to various regimes, one first needs to determine what regimes are. There are different approaches to establishing regimes. One way is to specify regimes based on knowledge and experience in the markets. For example, one might categorise market regimes using ‘boom’ and ‘bust’ cycles, periods of high or low equity market volatility. Changes in monetary policy, or ‘risk-on’ versus ‘risk-off’ sentiment, believing those to be good indicators of meaningfully changing market conditions.
An alternative, more data-driven approach is letting historical data on assets and/or market risks delineate the regimes for you. By using multiple machine learning models we try to cluster and classify regimes and analyse the impact of such regimes on assets or the broader market overall.
Identifying these regimes is useful for making broader asset universe changes for given conditions or making allocation decisions for a given portfolio, allowing us to help investors rebalance and keep their portfolio actively engaged toward achieving their goals.
The machine learning method can add value by letting a large amount of historical data determine the regimes for you. Labelling such conditions and analysing them against existing portfolios or stress testing against them allows us to build better asset allocation models for long-term investors.